The Ultimate Guide to Image Classification Annotation Tools
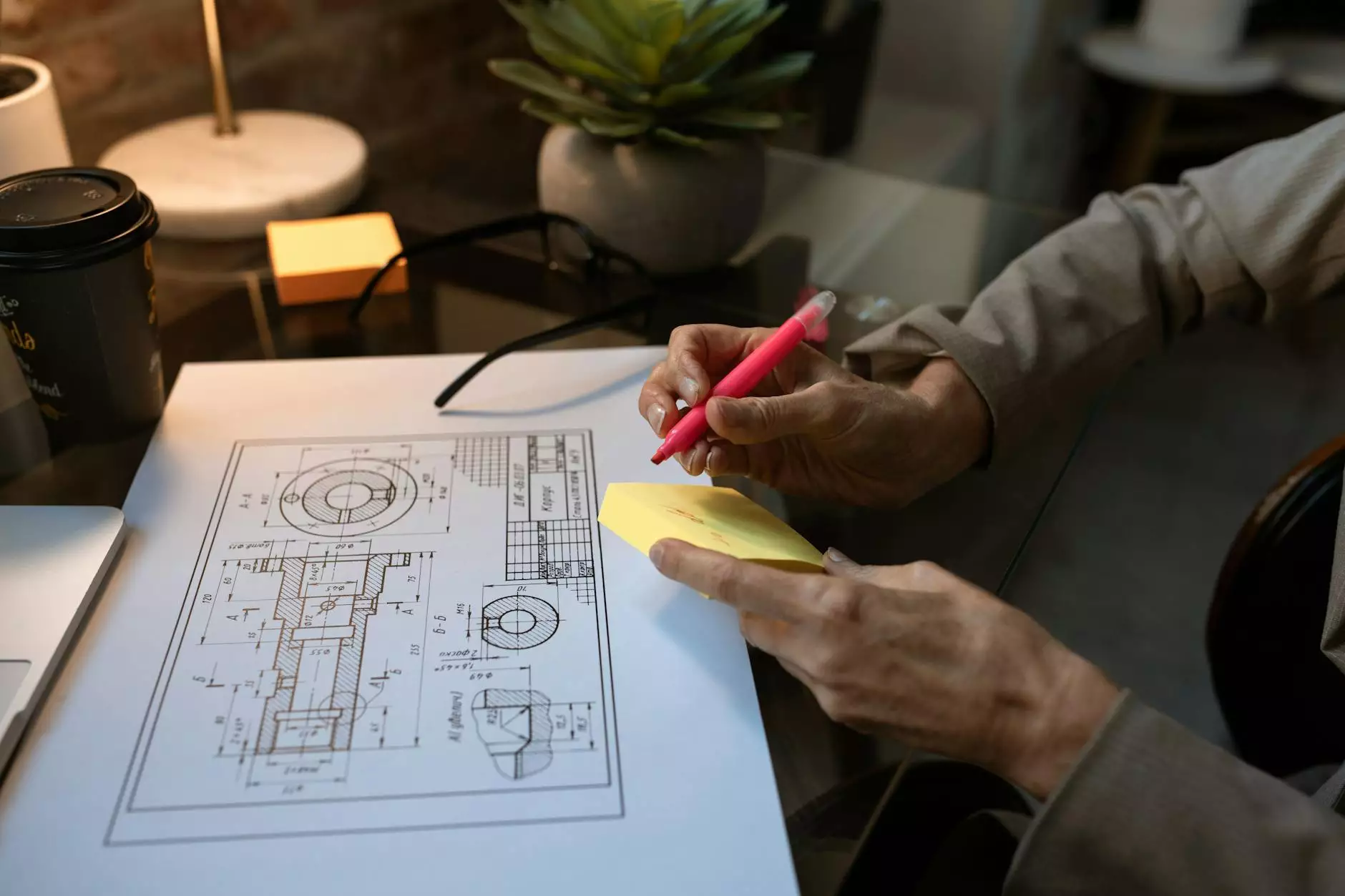
In the realm of business and technology, the development of sophisticated machine learning algorithms has transformed how we analyze and utilize data. One of the foundational aspects of this transformation is data annotation, specifically the use of image classification annotation tools. As businesses strive to leverage artificial intelligence (AI) and machine learning, understanding the nuances of these tools becomes essential for maintaining a competitive edge.
What Are Image Classification Annotation Tools?
Image classification annotation tools are software applications designed to label and categorize images to prepare datasets for training algorithms. These tools enable data scientists and machine learning engineers to create structured datasets that improve the quality and accuracy of AI models. Unlike traditional methods of data entry, these annotation tools allow for a more interactive and intuitive labeling process.
Importance of Data Annotation in Business
In today’s data-driven landscape, businesses generate and collect massive amounts of data. However, raw data is often unstructured and requires thorough annotation to be useful. Effective data annotation has numerous benefits:
- Enhanced Data Quality: Well-annotated data directly influences the effectiveness of AI models, leading to improved decision-making.
- Improved Model Accuracy: Properly labeled datasets enable models to learn and predict with greater precision.
- Faster Training Processes: Automation in annotation can significantly reduce the time required to prepare data.
- Competitive Advantage: Companies utilizing accurate data annotation can respond more effectively to market trends and consumer needs.
How Image Classification Annotation Tools Work
The operation of image classification annotation tools can be broken down into several key steps:
1. Data Input
The first step involves importing images into the tool. This can be done through various means including uploading files or integrating with cloud storage services.
2. Annotation Process
Once the images are uploaded, users can begin the annotation process. Common techniques used in image classification include:
- Bounding Boxes: Drawing squares or rectangles around objects of interest.
- Semantic Segmentation: Classifying individual pixels in an image to differentiate between object types.
- Polygon Annotation: Creating polygons around objects for more complex shapes.
- Image Tagging: Adding descriptive labels or tags to images for contextual understanding.
3. Quality Assurance
A crucial aspect of the annotation process is the quality assurance phase, where labeled data is reviewed for accuracy. This can involve multiple rounds of checking and verification to ensure the highest quality output.
Best Practices for Using Image Classification Annotation Tools
To maximize the effectiveness of image classification annotation tools, businesses should follow these best practices:
- Choose the Right Tool: Different projects may require different annotation capabilities. Research and select a tool that meets your specific needs.
- Establish Clear Guidelines: Provide annotators with clear instructions and guidelines to ensure consistency across the dataset.
- Incremental Training: Train your models incrementally as new data is annotated, allowing for continuous improvement.
- Regular Audits: Perform routine checks and audits on annotated data to maintain quality and relevance.
Features to Look for in an Image Classification Annotation Tool
When selecting an image classification annotation tool, consider the following features:
- User-Friendly Interface: An intuitive design simplifies the annotation process for both technical and non-technical users.
- Collaboration Capabilities: The ability for team members to work together on projects in real time increases efficiency.
- Integration Options: Ensure that the tool can be integrated with your existing workflow and data storage solutions.
- Automation Features: Tools that offer machine learning-assisted annotation can speed up the process and reduce human error.
The Role of Keylabs.ai in Data Annotation
At Keylabs.ai, we specialize in providing top-tier data annotation tools and platforms tailored for diverse business needs. We recognize that effective data annotation is pivotal to the success of machine learning initiatives. Our platform not only offers comprehensive data annotation tools but also features advanced functionalities like automation, real-time collaboration, and extensive customization options.
Our Data Annotation Services Include:
- Image Annotation: High-quality labeling for computer vision projects.
- Video Annotation: Detailed annotation for time-based data analysis.
- 3D Point Cloud Annotation: Specializing in autonomous vehicle data training.
- Text Annotation: Enhancing NLP projects with accurate text data labeling.
Case Studies: Successful Applications of Annotation Tools
1. Enhancing Retail Inventory Management
One leading retail chain leveraged image classification annotation tools to optimize inventory management. By accurately tagging product images, the machine learning model could identify stock levels more effectively, resulting in a 25% reduction in overstock and increased profitability.
2. Advancements in Healthcare
Healthcare providers have utilized image classification annotation tools for analyzing medical imaging data. By training AI systems to recognize signs of ailments in X-rays and MRIs, practitioners can diagnose patients faster and more accurately, ultimately improving patient outcomes.
Challenges and Solutions in Data Annotation
While the benefits of image classification annotation tools are clear, challenges do exist. Understanding these challenges and their solutions is key to optimizing your annotation strategy:
1. Scalability Issues
As data volumes grow, scaling annotation efforts can become complex. Solution: Invest in automation features within annotation tools to handle larger datasets more efficiently.
2. Quality Control
Ensuring consistent quality across a large annotated dataset can be challenging. Solution: Implement tiered review processes and use metrics to monitor accuracy levels.
The Future of Image Classification Annotation Tools
As technology evolves, so does the potential for image classification annotation tools. The future promises advancements in areas such as:
- AI-Driven Annotation: Tools will increasingly leverage AI to assist in the annotation process, thereby reducing the workload on human annotators.
- Real-Time Collaboration: Improved cloud-based solutions will allow teams to collaborate in real time, even from different locations.
- Expanded Automation Tools: Future tools will likely include advanced capabilities for automating repetitive tasks in the annotation process, making data preparation faster than ever before.
Conclusion
In conclusion, image classification annotation tools play a critical role in the successful implementation of machine learning and AI initiatives. Businesses that invest in high-quality data annotation platforms will find themselves better equipped to harness the power of their data, driving innovation and improvement across their operations. At Keylabs.ai, we are committed to providing exceptional data annotation tools that empower organizations to excel in their respective fields.
By understanding the importance of data quality and employing the right annotation strategies, businesses can significantly enhance their machine learning outcomes, ensuring they stay ahead in the competitive landscape of today’s technology-driven world.