The Vital Role of Labeling Training Data in Business Success
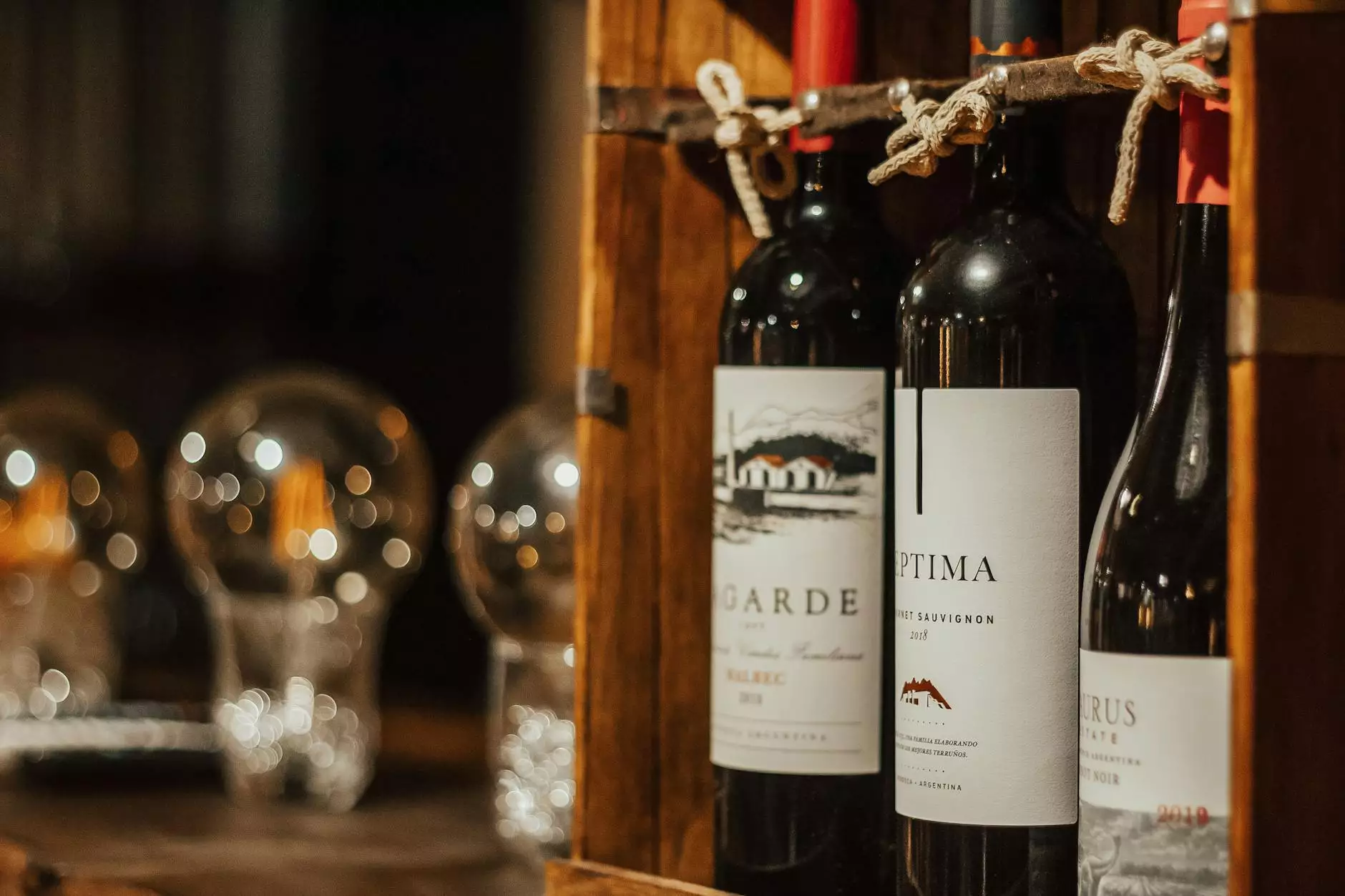
In today's digital landscape, the demand for high-quality data has never been greater. Businesses across various sectors are leveraging machine learning to gain insights, improve operations, and enhance customer experiences. A foundational element of successful machine learning applications is the process of labeling training data. This article explores what labeling training data entails, its significance to businesses, and how tools from Keylabs.ai can optimize your data annotation processes.
Understanding Labeling Training Data
Labeling training data refers to the process of tagging or annotating data sets to provide the necessary context for machine learning algorithms. This involves categorizing raw data so that the algorithm can learn and make predictions based on that data. Without proper labeling, even the most advanced algorithms can falter.
There are various forms of data that require labeling, including:
- Text Data: Sentiment analysis, keyword extraction, and entity recognition.
- Image Data: Object detection, image classification, and segmentation.
- Audio Data: Speech recognition and emotion detection.
- Video Data: Action recognition and scene segmentation.
The Significance of Accurate Labeling
Accurate labeling of training data is crucial for several reasons:
1. Enhances Model Accuracy
The primary objective of labeling is to train machine learning models effectively. High-quality labeled data leads to accurate predictions, which can significantly affect business outcomes.
2. Saves Time and Costs
Investing in effective labeling processes can save businesses time and resources. Automation tools and platforms provided by Keylabs.ai can help streamline the labeling process, reducing the need for extensive manual input.
3. Improves Decision Making
Businesses rely on insights derived from labeled data for strategic decision-making. When the data is well-labeled, the decisions made based on these insights are more likely to be effective and relevant.
Challenges in Labeling Training Data
Despite its importance, businesses often encounter several challenges in the data labeling process:
1. Volume and Variety of Data
The sheer amount of data generated can overwhelm organizations, especially if they are not equipped with the right tools to handle diverse data types.
2. Subjectivity in Labeling
Labeling data can sometimes require human judgment, which can introduce bias and inconsistencies. This is especially true in fields like sentiment analysis where the interpretation of data can vary across individuals.
3. Resource Intensive
Manual data labeling can be labor-intensive, often requiring specialized knowledge and prolonged effort, which can strain organizational resources.
Leveraging Data Annotation Tools
To overcome these challenges, businesses are increasingly turning to data annotation tools. These platforms provide streamlined solutions for labeling training data, ensuring consistency, speed, and accuracy. Here’s how Keylabs.ai stands out in this domain:
1. Automated Annotation
Keylabs.ai leverages advanced algorithms and machine learning models to automate the annotation process, dramatically speeding up the time it takes to label large datasets. This allows businesses to focus on analysis rather than data preparation.
2. Customizable Solutions
Different businesses have different requirements. Keylabs.ai offers customizable labeling solutions that adapt to the unique needs of any organization, whether it’s for healthcare data, retail insights, or any other sector.
3. Quality Assurance Mechanisms
Ensuring the quality of labeled data is imperative. Keylabs.ai implements rigorous quality assurance protocols, including double-validation and machine learning feedback mechanisms, to ensure accuracy and reliability.
The Process of Labeling Training Data
The process of labeling training data can be broken down into several key steps:
- Data Collection: Gathering raw data from various sources.
- Preprocessing: Cleaning and preparing data for annotation.
- Annotation: Tagging data with context-specific labels.
- Validation: Checking the quality and accuracy of labels.
- Integration: Incorporating labeled data into machine learning models.
Case Studies of Successful Data Annotation
Several organizations have successfully leveraged labeling training data to enhance their operations. Below are a few notable examples:
1. Healthcare Analytics
A leading healthcare provider used Keylabs.ai’s annotation platform to label patient data for predictive analytics. By accurately labeling medical records, they improved patient outcomes through better diagnosis and treatment strategies.
2. Retail Personalization
A major retailer employed data annotation tools to label customer interaction data. This led to personalized marketing efforts that significantly increased customer engagement and sales, illustrating the power of well-labeled training data.
3. Autonomous Vehicles
Companies in the automotive industry, particularly those working on autonomous vehicles, require massive amounts of labeled data to train their models. Using Keylabs.ai, one such company streamlined its data labeling efforts, resulting in a more efficient development cycle and faster deployment of self-driving technologies.
Future Trends in Data Annotation
The landscape of data annotation is continually evolving. Here are some anticipated trends that could shape how businesses approach labeling training data:
1. Increased Use of AI
Artificial intelligence is expected to play a larger role in automating data labeling processes, reducing the reliance on manual annotation and significantly improving efficiency.
2. Real-Time Annotation
As businesses gather data at unprecedented speeds, the demand for real-time annotation tools will grow, necessitating platforms that can keep up with the pace of data generation.
3. Higher Demand for Diverse Data Sets
With a focus on inclusivity and representation, the need for diverse and comprehensive data sets will drive businesses to adopt more rigorous data labeling practices.
Conclusion: The Path to Effective Data Labeling
Labeling training data is not merely a step in the machine learning process; it is a critical component that influences the overall efficacy of data-driven decisions in business. By prioritizing high-quality annotation, organizations can unlock the full potential of their data. Keylabs.ai offers innovative tools and solutions tailored to optimize your data labeling needs, ensuring that your business remains competitive in a rapidly changing digital landscape.
Investing in effective data labeling processes today will pave the way for smarter decision-making tomorrow. Explore how Keylabs.ai can transform your data annotation experience and lead your business to greater success.